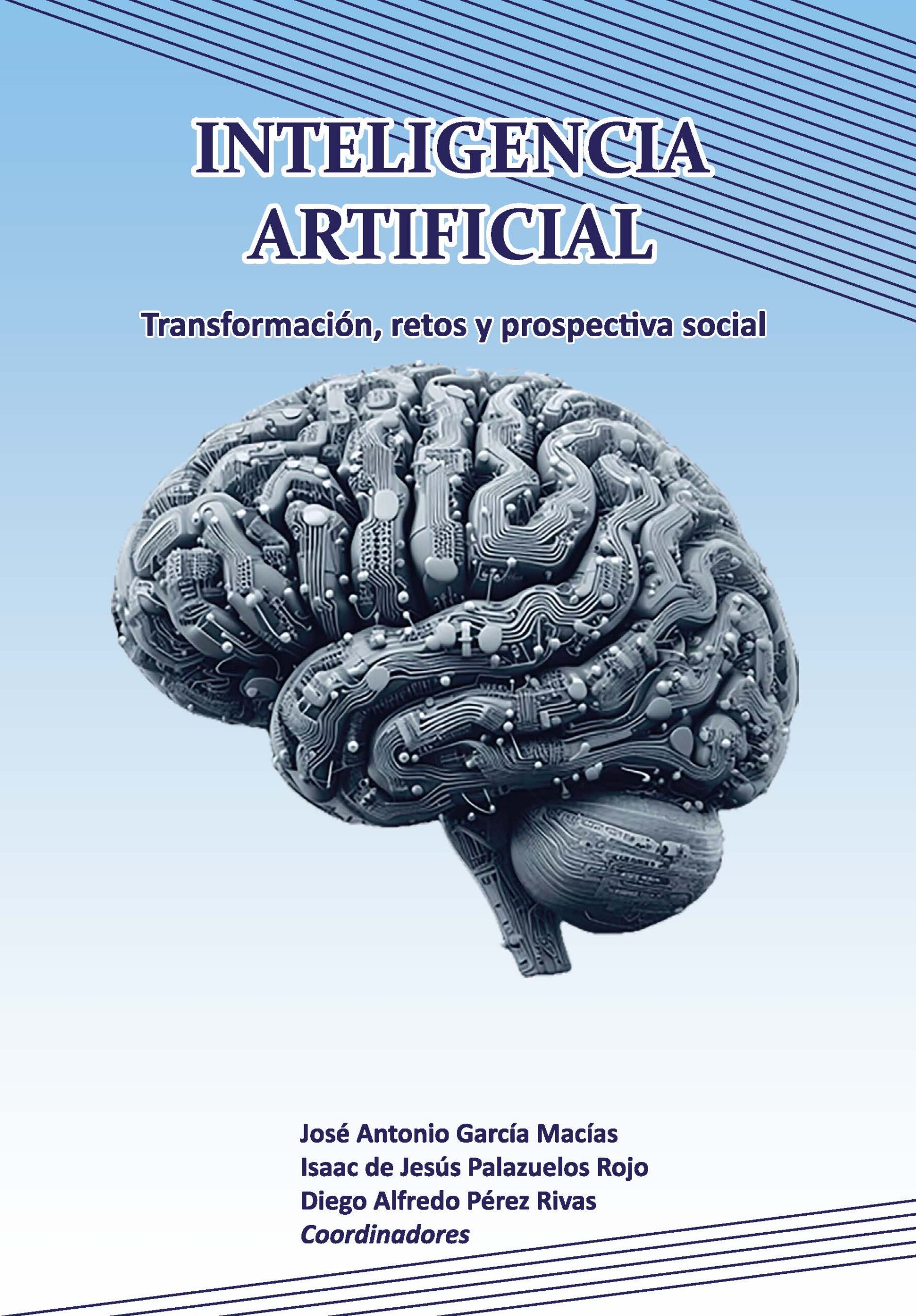
ISBN
Formato digital
978-84-10215-89-4
Fecha de publicación
14-11-2024
Licencia
D. R. © copyright 2024; José Antonio García Macías, Isaac de Jesús Palazuelos Rojo y Diego Alfredo Pérez Rivas.
Guillermo Hernández-Santana
Universidad Autónoma de Baja California
0000-0003-0367-357X
Irvin Hussein López-Nava
Centro de Investigación Científica y de Educación Superior de Ensenada
0000-0003-3979-9465
Acerca de
Es indudable que a partir del desarrollo de la inteligencia artificial (IA) y los sistemas de procesamiento de lenguaje natural (PLN), las computadoras comenzaron a comprender, interpretar y generar lenguaje humano en sus diversas formas, sin embargo, estos sistemas cuentan con un mayor desarrollo en relación con las lenguas orales que a las lenguas de modalidad visogestual. Esto responde a la colección de datos lingüísticos que contamos en la actualidad, la cual, es significativamente mayor en lenguas orales que señadas. Es decir, que el desarrollo de la IA y del PLN tiene en la actualidad avances importantes en cuanto a la capacidad de las máquinas para interpretar y generar texto en diversos contextos, desde traductores automáticos hasta asistentes virtuales, pasando por chatbots.
En este capítulo discutimos la importancia del reconocimiento auto-mático de las lenguas de modalidad visogestual, vital para la inclusión y comunicación efectiva de las personas sordas. En primer lugar, estos sistemas representan un avance tecnológico significativo en el campo de la accesibilidad, permitiendo una mayor autonomía y participación de este sector de la población en diversos contextos sociales y profesionales. Algunos de los beneficios de estas aplicaciones y su implementación pueden repercutir en tecnologías de reconocimiento automático de lenguas de señas las cuales pueden facilitar la comunicación entre personas sordas y oyentes, reduciendo las barreras lingüísticas y promoviendo la inclusión social, entre otros beneficios.
Referencias
Albanie, S. et al. (2020). BSL-1K: Scaling Up Co-articulated Sign Language Recognition Using Mouthing Cues. En Vedaldi, A., Bischof, H., Brox, T., Frahm, J. M. (Eds.), Computer Vision – ECCV 2020. ECCV 2020. Lecture Notes in Computer Science (Vol. 12356). Springer, Cham. https://doi.org/10.1007/978-3-030-58621-8_3
Aly, S. y Aly, W. (2020). DeepArSLR: A novel signer-independent deep learning framework for isolated Arabic sign language gestures recognition. IEEE access: practical innovations, open solutions, 8, 83199-83212. https://doi.org/10.1109/access.2020.2990699
Agarwal, P., Liao, J., Hooper, S., & Sperling, R. (2021). Applying deep learning to a sign language progress monitoring system. Distance Learning, 18(3), 45-55. [https://eric.ed.gov/?q=artifical+intelligence+AND+sign+language+&ff1=subArtificial+Intelligence&ff2=dtySince_2020&id=EJ1327302]
Bragg, D., Koller, O., Bellard, M., Berke, L., Boudreault, P., Braffort, A., Caselli, N., Huenerfauth, M., Kacorri, H., Verhoef, T., Vogler, C. y Ringel Morris, M. (2019). Sign language recognition, generation, and translation: An interdisciplinary perspective. The 21st International ACM SIGACCESS Conference on Computers and Accessibility.
Camgoz, N.C.; Hadfield, S.; Koller, O.; Ney, H.; Bowden, R. (2018). Neural sign language translation. En Proceedings of the IEEE Conference on Computer Vision and Pattern Recognition. Salt Lake City, UT, USA.
Camgoz, N. C., Hadfield, S., Koller, O. y Bowden, R. (2017). SubUNets: End-to-end Hand Shape and Continuous Sign Language Recognition. *ICCV.*
Elatawy, S. M., Hawa, D. M., Ewees, A. A. y Saad, A. M. (2020). Recognition system for alphabet Arabic sign language using neutrosophic and fuzzy c-means. Education and Information Technologies, 25(6), 5601–5616. https://doi.org/10.1007/s10639-020-10184-6
Forster, J.; Schmidt, C.; Koller, O.; Bellgardt, M.; Ney, H. (2014). Extensions of the Sign Language Recognition and Translation Corpus RWTH-PHOENIX-Weather. En Proceedings of the LREC, (pp. 1911–1916).Reykjavik, Iceland.
Fridman-Mintz, B. (2005). LSM tense & aspect inflections. A Dissertation Submitted to the Faculty of the Graduate School of Arts and Sciences of Georgetown University. https://www.academia.edu/59937562/LSM_tense_and_aspect_inflections
Galicia, R., Carranza, O., Jiménez, E. D, Rivera, G. E. (2015). Mexican sign language recognition using movement sensor (pp. 573-578).
Garcia-Bautista, G., Trujillo-Romero, F., y Caballero-Morales, S. (2017). Mexican sign language recognition using kinect and data time warping algorithm. 2017 International Conference On Electronics, Communications And Computers (CONIELECOMP). https://doi.org/10.1109/conielecomp.2017.7891832
Gellai, D. B. (2023). Enterprising academics: Heterarchical policy networks for artificial intelligence in British higher education. ECNU Review of Education, 6(4), 568–596. [http://files.eric.ed.gov/fulltext/EJ1401707.pdf]
Gortarez-Pelayo, J. J., Morfín-Chávez, R. F., & Lopez-Nava, I. H. (2023, noviembre). DAKTILOS: An Interactive Platform for Teaching Mexican Sign Language (LSM). En International Conference on Ubiquitous Computing and Ambient Intelligence (pp. 264-269). Cham: Springer Nature Switzerland.
Herrera Crespo, J. (2021). Diseño y validación de un software de reconocimiento de gestos sirviéndose del acelerómetro de un reloj inteligente. Universitat Politècnica de València. http://hdl.handle.net/10251/172272
INALSA (s/f). Proyecto de Ley de Reconocimiento de la Lengua de Señas Argentina – LSA, y de creación del Instituto Nacional de Lenqua de Señas Argentina. [https://inalsa.cas.org.ar/nuestra-lsa/la-ls-en-el-mundo/#:~:text=Ethonogue%2C%20una%20enciclopedia%20que%20cataloga,señas%20dispersas%20alrededor%20del%20mundo.]
Kothadiya, D., Bhatt, C., Sapariya, K., Patel, K., Gil, A., & Corchado Rodríguez, J. (2022). Deepsign: Sign Language Detection and Recognition Using Deep Learning. Electronics, 11, 1780. https://doi.org/10.3390/electronics11111780
Koller, O., Zargaran, S. y Ney, H. (2015). Continuous sign language recognition: Towards large vocabulary statistical recognition systems handling multiple signers. Computer Vision and Image Understanding, 141, 108-125.
Lang, H., Manganelli, J., & Panchanathan, S. (2020). Virtual reality and augmented reality in social learning spaces for people with visual impairments. IEEE Transactions on Learning Technologies, 13(2), 422-433.
Liang, X., Angelopoulou, A., Kapetanios, E., Woll, B., Al Batat, R., & Woolfe, T. (2020). A multi-modal machine learning approach and toolkit to automate recognition of early stages of dementia among british sign language users. En Computer Vision–ECCV 2020 Workshops: Glasgow, UK, August 23–28, 2020, Proceedings, Part II 16 (pp. 278-293). Springer International Publishing.
Martínez-Gutiérrez, M. E., Rojano-Cáceres, J. R., Benítez-Guerrero, E., y Sánchez-Barrera, H. E. (2019). Data acquisition software for sign language recognition. Res. Comput. Sci., 148(3), 205–211
Mejía-Peréz, K., Córdova-Esparza, D.-M., Terven, J., Herrera-Navarro, A.-M., García-Ramírez, T. y Ramírez-Pedraza, A. (2022). Automatic recognition of Mexican Sign Language using a depth camera and recurrent neural networks. Applied Sciences (Basel, Switzerland), 12(11), 5523. https://doi.org/10.3390/app12115523
Moher, David, Shamseer, Larissa, Clarke, Mike, Ghersi, Davina, Liberati, Alessandro, Petticrew, Mark, Shekelle, Paul, & Stewart, Lesley A. (2016). Ítems de referencia para publicar Protocolos de Revisiones Sistemáticas y Metaanálisis: declaración PRISMA-P 2015. Revista Española de Nutrición Humana y Dietética, 20(2), 148-160. https://dx.doi.org/10.14306/renhyd.20.2.223
Morfín-Chávez, R. F., Gortarez-Pelayo, J. J., & Lopez-Nava, I. H. (2023). Fingerspelling Recognition in Mexican Sign Language (LSM) Using Machine Learning. En Mexican International Conference on Artificial Intelligence (pp. 110-120). Cham: Springer Nature Switzerland.
Murakami, K., & Taguchi, H. (1991). Gesture recognition using recurrent neural networks. Proceedings of the SIGCHI Conference on Human Factors in Computing Systems.
Ordaz-Hernández, K., Castillo-Gaytán, D., Rodríguez-Recio, A. S., Boone-Obregón, R. D., Hernández-García, L. Á., & Hilario-Acuapan, G. (2023). Hacia la construcción de una base de datos abierta de la LSM. Pädi Boletín Científico de Ciencias Básicas e Ingenierías del ICBI, 11. (Especial 2), 134-141.
Ompi. (2019). BrightSign: el guante inteligente que da voz a quienes no pueden hablar. (s. f.). Wipo.int. Recuperado el 5 de febrero de 2024, de https://www.wipo.int/wipo_magazine/es/2019/05/article_0005.html
Papastratis, I., Chatzikonstantinou, C., Konstantinidis, D., Dimitropoulos, K., & Daras, P. (2021). Artificial intelligence technologies for sign language. Sensors (Basel, Switzerland), 21(17), 5843. https://doi.org/10.3390/s21175843
Pigou, L., Dieleman, S., Kindermans, P. J., & Schrauwen, B. (2015). Sign language recognition using convolutional neural networks. Computer Vision and Pattern Recognition Workshops.
Quandt, L. (2020). Teaching ASL Signs using Signing Avatars and Immersive Learning in Virtual Reality. The 22nd International ACM SIGACCESS Conference on Computers and Accessibility. doi:10.1145/3373625.3418042
Rios-Figueroa, H. V., Sánchez-García, A. J., Sosa-Jiménez, C. O., & Solís-González-Cosío, A. L. (2022). Use of spherical and Cartesian features for learning and recognition of the static Mexican Sign Language alphabet. Mathematics, 10(16), 2904. https://doi.org/10.3390/math10162904
Schioppo Zachary Meyer Deigo Fabiano Shaun Canavan. (2019). Learning Sign Language in a Virtual Environment. En Proceedings of ACM CHI conference, Glasgow, UK, May 2019 (CHI ’19), 6 pages. https://doi.org/10.475/123_4
Solís, F. J., Toxqui, C., & Martinez, D. (2015). Mexican Sign Language Recognition Using Jacobi-Fourier Moments.
Solis, F. J., Martinez, D., & Espinoza, O. (2016). Automatic Mexican Sign Language Recognition Using Normalized Moments and Artificial Neural Networks.
Solís-V., J.-F., Toxqui-Quitl, C., Martínez-Martínez, D. y Margarita H.-G. (2014). Mexican sign language recognition using normalized moments and artificial neural networks.
Sosa-Jimenez, C., Rios-Figueroa, H., Rechy-Ramirez, E., Marin-Hernandez, A., y Gonzalez-Cosio, A. (2017). Real-time Mexican Sign Language recognition. IEEE International Autumn Meeting On Power, Electronics And Computing (ROPEC). https://doi.org/10.1109/ropec.2017.8261606
Sosa-Jimenez, C. O., Rios-Figueroa, H., & Ana Luisa Solís-González-Cosío. (2022). A Prototype for Mexican Sign Language Recognition and Synthesis in Support of a Primary Care Physician.
Sturman, D. J., & Zeltzer, D. (1994). A Survey of Glove-Based Input. IEEE Computer Graphics and Applications, 14(1), 30-39.
Sreemathy, R., Turuk, M., Kulkarni, I. y Khurana, S. (2023). Sign language recognition using artificial intelligence. Education and Information Technologies, 28(5), 5259–5278. (https://doi.org/10.1007/s10639-022-11391-z]
Sugandhi, P. Kumar y Kaur, S. (2021). Indian Sign Language Generation System. En Computer, 54(3), 37-46. doi: 10.1109/MC.2020.2992237.
Varela-Santos, H., Morales-Jiménez, A., Córdova-Esparza, D. M., Terven, J., Mirelez-Delgado, F. D. y Orenday-Delgado, A. (2021). Assistive device for the translation from mexican sign language to verbal language. Computación y Sistemas, 25(3), 451-464.