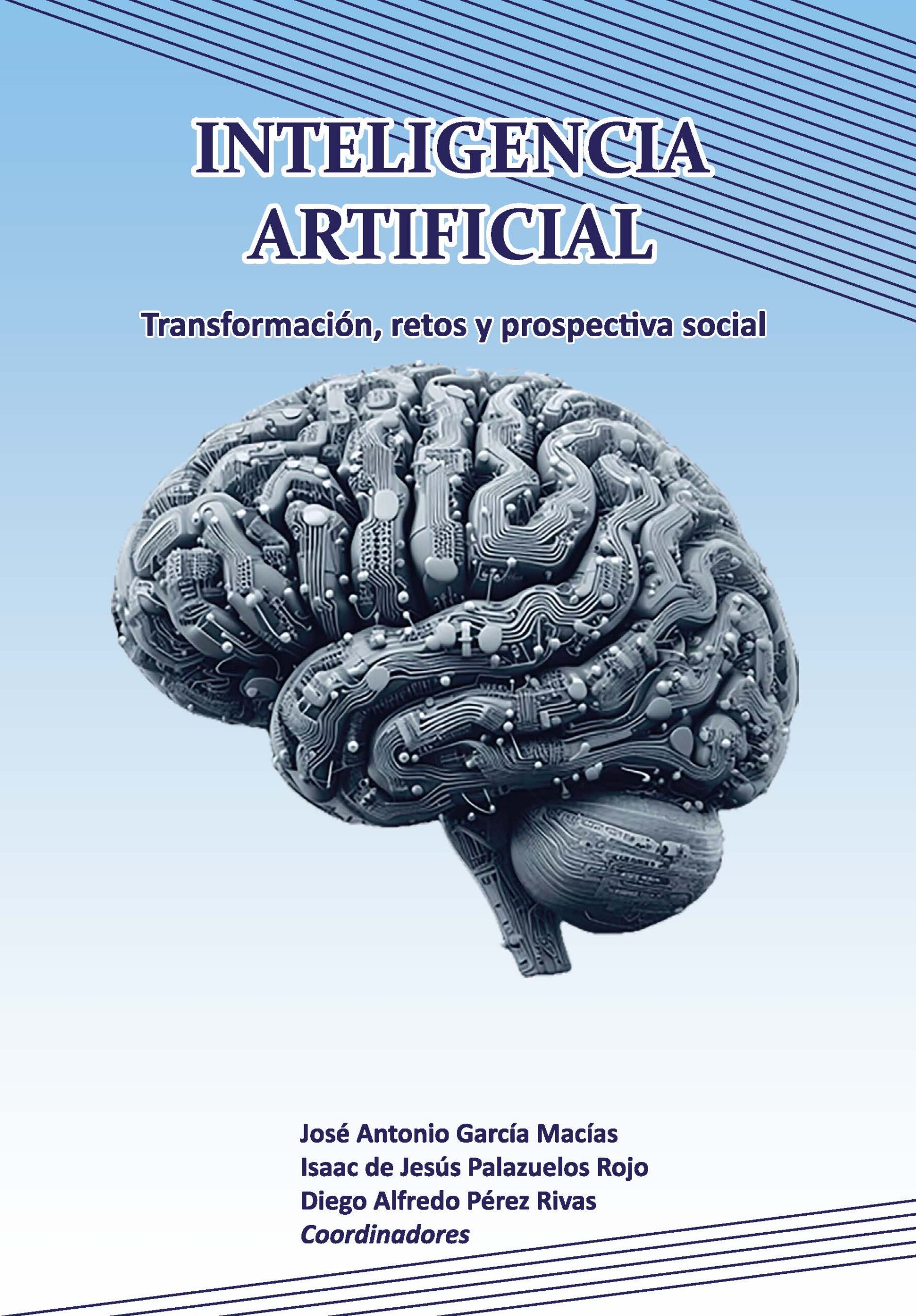
ISBN
Formato digital
978-84-10215-89-4
Fecha de publicación
14-11-2024
Licencia
D. R. © copyright 2024; José Antonio García Macías, Isaac de Jesús Palazuelos Rojo y Diego Alfredo Pérez Rivas.
Oscar Méndez García
Universidad Autónoma de Baja California
0009-0006-6862-9122
Acerca de
El sector agrícola es de gran importancia para todos los países. Con el incremento de la población se espera que la demanda alimenticia aumente de manera considerable para el año 2050 (Shariff et al., 2022). Por lo mismo, la producción de este sector es un tema relevante, en virtud de todos los bienes que se producen en las actividades agrícolas, que va desde la preparación de la tierra, cuidado de semillas, cultivos de alimentos, proceso de cosecha, uso de suelo y agua, así como el impacto en el medioambiente. La gestión eficiente de estos recursos ha provocado una transformación tecnológica del sector agrícola con la finalidad de incrementar la producción, disminuir los costos y responder a la alta demanda de manera sostenible con los recursos disponibles.
Con el tiempo, el sector agrícola ha evolucionado adaptándose a los diversos cambios que se han presentado, partiendo de su forma tradicional hasta llegar a la automatización de sus procesos buscando la mejora continua. Es por ello por lo que en la actualidad, este sector ha puesto su interés en la tecnología como fuente primaria para generar innovación, encontrando diferentes aplicaciones para el uso de tecnologías digitales que se pueden enfocar en el sector alimentario, como son drones, vigilancia satelital, tractores de especialización, tecnologías inteligentes, internet de las cosas (IoT), big data y blockchain. Debido a la importancia del sector agrícola, no solamente como impulsor económico sino también por su enorme aportación al sector alimentario (Suarez-Guzmán et al., 2020).
Referencias
Adli, H. K., Remli, M. A., Wan Salihin Wong, K. N. S., Ismail, N. A., González-Briones, A., Corchado, J. M., & Mohamad, M. S. (2023). Recent Advancements and Challenges of AIoT Application in Smart Agriculture: A Review. Sensors, 23(7), 3752. https://doi.org/10.3390/s23073752
Ang, K. L.-M. y Seng, J. K. P. (2021). Big Data and Machine Learning With Hyperspectral Information in Agriculture. IEEE Access, 9, 36699–36718. https://doi.org/10.1109/ACCESS.2021.3051196
Ayoub Shaikh, T., Rasool, T., & Rasheed Lone, F. (2022). Towards leveraging the role of machine learning and artificial intelligence in precision agriculture and smart farming. Computers and Electronics in Agriculture, 198, 107-119. https://doi.org/10.1016/j.compag.2022.107119
Bhat, S. A., & Huang, N.-F. (2021). Big Data and AI Revolution in Precision Agriculture: Survey and Challenges. IEEE Access, 9, 110209–110222. https://doi.org/10.1109/ACCESS.2021.3102227
Ci̇Velek, Ç. (2021). Development of an IoT based (LoRaWAN) Tractor Tracking System. Tarım Bilimleri Dergisi. https://doi.org/10.15832/ankutbd.769200
Cook, P., & O’Neill, F. (2020). Artificial Intelligence in Agribusiness is Growing in Emerging Markets. https://policycommons.net/artifacts/1279703/artificial-intelligence-in-agribusiness-is-growing-in-emerging-markets/1870529/
Dakshayini, M. y Balaji Prabhu, B. V. (2020). An Effective Big Data and Blockchain (BD-BC) Based Decision Support Model for Sustainable Agriculture System. En A. Haldorai, A. Ramu, S. Mohanram, & C. C. Onn (Eds.), EAI International Conference on Big Data Innovation for Sustainable Cognitive Computing (pp. 77–86). Springer International Publishing. https://doi.org/10.1007/978-3-030-19562-5_8
Idarraga Vargas, A., Pinzon Gonzalez, J. E., & Vergara Suarez, S. D. J. (2024). Análisis de los costos de la aplicación de drones en la fumigación en las fincas bananeras del municipio de Apartadó. Estudio de caso. https://repository.ucc.edu.co/entities/publication/1fe239ef-e6d0-4a3b-810d-daeecd5c17e0
Kamilaris, A., Kartakoullis, A., & Prenafeta-Boldú, F. X. (2017). A review on the practice of big data analysis in agriculture. Computers and Electronics in Agriculture, 143, 23-37. https://doi.org/10.1016/j.compag.2017.09.037
Kim, N., Na, S.-I., Park, C.-W., Huh, M., Oh, J., Ha, K.-J., Cho, J., & Lee, Y.-W. (2020). An Artificial Intelligence Approach to Prediction of Corn Yields under Extreme Weather Conditions Using Satellite and Meteorological Data. Applied Sciences, 10(11), 3785. https://doi.org/10.3390/app10113785
Linaza, M. T., Posada, J., Bund, J., Eisert, P., Quartulli, M., Döllner, J., Pagani, A., G. Olaizola, I., Barriguinha, A., Moysiadis, T., & Lucat, L. (2021). Data-Driven Artificial Intelligence Applications for Sustainable Precision Agriculture. Agronomy, 11(6), 1227. https://doi.org/10.3390/agronomy11061227
Maya Gopal P.S., & Chintala, B. R. (2020). Big Data Challenges and Opportunities in Agriculture: International Journal of Agricultural and Environmental Information Systems, 11(1), 48–66. https://doi.org/10.4018/IJAEIS.2020010103
Misra, N. N., Dixit, Y., Al-Mallahi, A., Bhullar, M. S., Upadhyay, R., & Martynenko, A. (2022). IoT, Big Data, and Artificial Intelligence in Agriculture and Food Industry. IEEE Internet of Things Journal, 9(9), 6305–6324. https://doi.org/10.1109/JIOT.2020.2998584
Nie, J., Wang, Y., Li, Y., & Chao, X. (2022). Artificial intelligence and digital twins in sustainable agriculture and forestry: A survey. Turkish Journal of Agriculture and Forestry, 46(5), 642–661. https://doi.org/10.55730/1300-011X.3033
Oliveira, R. C. D., & Silva, R. D. D. S. E. (2023). Artificial Intelligence in Agriculture: Benefits, Challenges, and Trends. Applied Sciences, 13(13), 7405. https://doi.org/10.3390/app13137405
Paredes, M., Zúñiga, W., Morocho Caiza, A. F., & Mendoza, M. (2021). Agricultura de precisión mediante WSN con nodos inteligentes aplicada a un sistema de riego en cultivo de mora. Revista Perspectivas, 3(2), 26–30. https://doi.org/10.47187/perspectivas.vol3iss2.pp26-30.2021
Raj, V. H. A., & De Carvalho, C. X. (2023). A Perspective on the Application of Artificial Intelligence in Sustainable Agriculture with Special Reference to Precision Agriculture. SDMIMD Journal of Management, 1–13. https://doi.org/10.18311/sdmimd/2023/33006
Ruiz-Real, J. L., Uribe-Toril, J., Torres Arriaza, J. A., & De Pablo Valenciano, J. (2020). A Look at the Past, Present and Future Research Trends of Artificial Intelligence in Agriculture. Agronomy, 10(11), 1839. https://doi.org/10.3390/agronomy10111839
Ryan, M., Isakhanyan, G., & Tekinerdogan, B. (2023). An interdisciplinary approach to artificial intelligence in agriculture. NJAS: Impact in Agricultural and Life Sciences, 95(1), 2168568. https://doi.org/10.1080/27685241.2023.2168568
Sood, A., Sharma, R. K., & Bhardwaj, A. K. (2022). Artificial intelligence research in agriculture: A review. Online Information Review, 46(6), 1054–1075. https://doi.org/10.1108/OIR-10-2020-0448
Subeesh, A., & Mehta, C. R. (2021). Automation and digitization of agriculture using artificial intelligence and internet of things. Artificial Intelligence in Agriculture, 5, 278–291. https://doi.org/10.1016/j.aiia.2021.11.004
Unal, Z. (2020). Smart Farming Becomes Even Smarter With Deep Learning—A Bibliographical Analysis. IEEE Access, 8, 105587–105609. https://doi.org/10.1109/ACCESS.2020.3000175
Van Der Merwe, D., Burchfield, D. R., Witt, T. D., Price, K. P., & Sharda, A. (2020). Drones in agriculture. En Advances in Agronomy (Vol. 162, pp. 1–30). Elsevier. https://doi.org/10.1016/bs.agron.2020.03.001
Vite Cevallos, H., Townsend Valencia, J., Carvajal Romero, H., Vite Cevallos, H., Townsend Valencia, J., & Carvajal Romero, H. (2020). Big Data e internet de las cosas en la producción de banano orgánico. Revista Universidad y Sociedad, 12(4), 192–200.
Wang, J., Peng, J., Li, H., Yin, C., Liu, W., Wang, T., & Zhang, H. (2021). Soil Salinity Mapping Using Machine Learning Algorithms with the Sentinel-2 MSI in Arid Areas, China. Remote Sensing, 13(2), 305. https://doi.org/10.3390/rs13020305
Yang, L. B. (2020). Application of Artificial Intelligence in Electrical Automation Control. Procedia Computer Science, 166, 292–295. https://doi.org/10.1016/j.procs.2020.02.097
Zhu, Zhou, Gao, Bao, He, & Feng. (2019). Near-Infrared Hyperspectral Imaging Combined with Deep Learning to Identify Cotton Seed Varieties. Molecules, 24(18), 3268. https://doi.org/10.3390/molecules24183268